Strategy In AI's Shifting Sands: Decoding Dynamics for Builders and Businesses
Oct 24, 2024
The AI landscape is a dynamic and competitive arena where international players are vying for dominance and also forming unlikely alliances that are driving market leadership. In Part One of this series, we explored these shifting dynamics, focusing on how the GCC - especially the UAE and Saudi Arabia - is emerging as a global hub for AI innovation. Click here to read Part One.
In Part Two, we delve into the implications of these developments, providing competitive insights for regional founders and thought-provoking considerations for enterprise leaders.
Coopetition. Why Regional Founders Must Unleash Its Untapped Power.
“Coopetition” is a highly relevant trend in the age of AI and GenAI, as players realize they need to rely more on each other. As a business strategy, coopetition involves forming strategic alliances to leverage complementary strengths, share resources, and achieve common goals, while still maintaining competitive aspects in other areas. While coopetition is necessary today, it will eventually give way to intense competition as companies develop their own capabilities. This will be a long marathon, and with big tech companies now operating with startup-like agility, maintaining a lead will prove more challenging than gaining one.
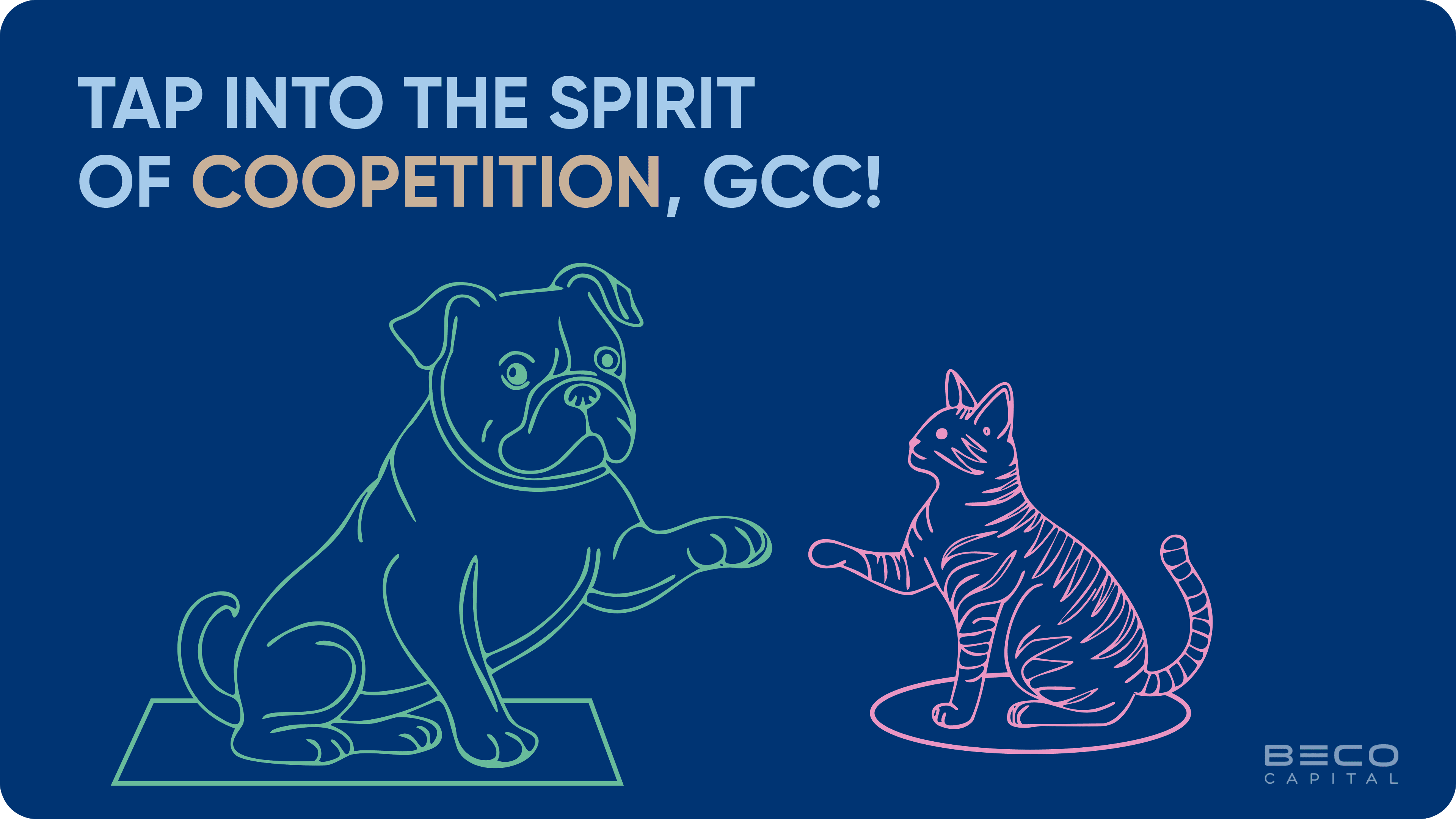
The implications are significant for global AI companies but particularly so for founders in our region. Despite the regional tech and venture capital ecosystem flourishing in recent years, a glaring lack of cooperation and coopetition among founders persists, making it hard for their businesses to scale efficiently. This inflates customer acquisition and retention costs, leading to unsustainable competition among numerous companies targeting the same customers.
We have long advocated for mid-market consolidation, yet a cultural shift towards genuine collaboration among founders is still needed, though many founders still resist this concept. In the context of AI, at the sovereign level we have seen large-scale B2G and B2B partnerships with specific entities in the UAE and KSA. Such partnerships have paved the way for excellence and rapid innovation, and we will showcase these in the next piece of our four-part series. For AI-first companies to flourish in the region, a mindset of coopetition must become part of the cultural norm among founders.
AI Is Going Mainstream Before Product-Market-Fit. What Does This Mean Today?
AI's mainstream adoption, while exciting, has also brought about significant implications for critical strategic decisions. The sheer velocity of innovation has led to considerable uncertainty for companies and founders alike. In response to this complex and metamorphic landscape, we've identified three competitive considerations that we believe are worth spotlighting.
Competitive Consideration 1: Build Where It Matters
In periods of slow technological evolution, building new products, features, or platforms in-house often made more sense than buying existing solutions. This was because specific capabilities and innovations were typically unavailable on the market, and there was no immediate threat of disruption. However, the advent of GenAI and LLMs has fundamentally altered this landscape, turning the traditional buy vs. build decision on its head. With the rapid advancements in AI, exemplified by each iteration of models like GPT from OpenAI, technological capabilities are evolving at a breakneck pace. This acceleration makes it crucial for product teams, tech leaders, C-suite executives, and founders to continuously reevaluate their strategies. The threat of imminent disruption is now a constant.

Strategic Implication
This accelerated pace of innovation means that the concept of buy vs. build should be at the forefront of every tech entrepreneur's mind. Companies that choose to build their own AI capabilities may struggle to keep up with the rapid advancements and scalability offered by specialized AI providers. Conversely, those that opt to integrate cutting-edge AI solutions, like GPT-4o, can rapidly enhance their products and services, staying ahead of competitors who may be slower to adopt. For instance, integrating pre-built AI solutions can provide immediate access to sophisticated natural language processing, enabling features such as advanced customer support chatbots, personalized marketing, and automated content creation without the time and resource investment required to develop these capabilities internally. As such, the ability to make informed buy vs. build decisions is now a critical factor in maintaining competitive advantage in the fast-evolving tech landscape driven by GenAI and LLMs.
We categorize this decision-making framework into two primary approaches:
Inward Focus: Leveraging GenAI internally to enhance operational efficiency, drive revenue growth, and optimize costs
Outward Focus: Empowering clients to adopt an inward focus by providing them with GenAI-powered solutions.
The breakneck speed of AI innovation cycles presents a significant challenge for enterprises today. IT teams and CIOs, who typically plan three to five years ahead, find their traditional procurement cycles disrupted by the swift advancements in Generative AI. There's a growing concern that products or solutions purchased or renewed today may become obsolete within just two years. This creates a dilemma: organizations need to invest in AI capabilities to stay competitive, but they also need assurance that their investments won't be rendered irrelevant by the next paradigm shift in AI technology. Consequently, decision-makers are increasingly cautious about allocating additional spend, seeking solutions that can adapt to the evolving AI landscape and provide long-term value despite the rapid pace of change.
Competitive Consideration 2: Get Your Data House In Order
The pace of improvements in LLMs is slowing as companies exhaust all the readily available data on the entire Internet, resulting in models and applications becoming more commoditized over time. Hence the need for proprietary data, which companies are now willing to pay a premium for. According to the IDC, only one to two percent of the world's data is accessible on the Internet, so accessing the remaining percentage is critical to enhancing the intelligence of such systems and AI as a whole. As a result, OpenAI and others have signed numerous multi-year agreements with groups such as News Corp, Associated Press, Reddit and Axel Springer to access their current and archived content to continue training their models to improve. Others, such as Blackrock, are acquiring alternative markets data providers, exemplified by their recent $3.2b purchase of Preqin.
With all LLM players racing towards Artificial General Intelligence (AGI), accessing deeper datasets is of the utmost importance. A new emerging trend, which has drawn its fair share of criticism, is the use of synthetic data to augment the training of models. On the one hand, you get the benefit of cost efficiencies, the ability to simulate rare or extreme scenarios that are considered edge cases and the broadening of available datasets. On the other hand, bias and hallucinations are typically much higher with synthetic data and the edge cases might be so unrealistic that it would not have any implications in the real world. Builders should always explore new data sources, including synthetic data, but an overreliance on this might end up significantly degrading the quality of models.
Proprietary data is a cornerstone of AI success. The quality and uniqueness of data inputs critically impact the quality of AI outputs. Proprietary data allows AI systems to generate more precise insights, tailor solutions to specific needs, and maintain a competitive edge by leveraging information that is not accessible to others. This advantage becomes crucial as businesses strive to harness AI's potential to drive innovation and efficiency. While proprietary data has always been crucial for data science and predictive modeling, the rise of GenAI has amplified its importance. Unlocking the value of proprietary data suddenly became the highest priority for an increasing number of organizations.

Strategic Implication
Companies of all sizes, especially smaller and younger ones, should adopt a data-driven approach to build out their capabilities and harness the power of the data they already possess. Whilst many of those companies might not view their data as core, ensuring that they are able to transform it from “dark data” (collected but unused information) into actionable insights will give them optionality for the future.
At BECO Capital, we recognize the strategic importance of proprietary data. With 12 years of experience, we are well-positioned to properly leverage this asset. In 2022, we made the conscious decision of collecting and organizing our structured and unstructured data, with ongoing data acquisition. While we are still in the process of determining the full scope of applications for our proprietary data, our early efforts underscore our commitment to leveraging this asset to its fullest potential.
Competitive Consideration 3: AI Is Redefining How You Price and Profit. Be Ready, Be Responsive.
The evolution of revenue models in software has seen significant changes over the decades, driven by technological advancements and shifting customer expectations. Initially, software companies predominantly operated on a license-based model, which gained prominence in the 1980s and 1990s. Customers paid a one-time fee for perpetual use of the software, which was common in the early days of enterprise software when solutions were installed on-premises and required substantial upfront investment. Companies like Microsoft (with Windows and Office) and Oracle (with its database software) were key examples of this model.
As technology progressed and the Internet became more ubiquitous in the 2000s, the subscription model emerged, popularized by SaaS companies. This model provided customers with ongoing access to software for a recurring fee, typically monthly or annually, enhancing affordability and scalability. Salesforce and Adobe are prominent examples of companies that successfully transitioned to this model.
More recently, in the 2010s, consumption-based pricing gained traction, where customers are charged based on their actual usage of the software, offering greater flexibility and aligning costs with value received. This model is often used by Cloud Service Providers (CSPs) as well as cloud observability (monitoring, analyzing and troubleshooting) and infrastructure companies, where users pay for the services they consume.
Strategic Implication
With AI, we anticipate another significant shift in revenue models, potentially moving towards outcome or savings-based pricing. We are intrigued by this shift and have discussed it extensively within our network over the last several months. AI can deliver immediate and measurable benefits, such as cost savings, increased revenues, and improved efficiencies, directly tied to the value it provides. This approach mirrors the business model seen in the solar industry, where vendors often share in customer savings. For example, SolarCity (now part of Tesla) might install panels at no upfront cost and then share in the energy savings realized by the customer over time. This model gained popularity in the late 2010s and early 2020s as renewable energy solutions became more widespread.

Similarly, AI companies could charge customers based on tangible outcomes. For instance, if an AI solution reduces operational costs by 20%, the provider could receive a percentage of those savings as payment. This incentivizes them to deliver real value and align their success with their customers. Moreover, it also accelerates customers payback, offering immediate benefits without the need for large upfront investments. This innovative revenue model could redefine how technology companies monetize their products, ensuring that the benefits of AI are accessible and impactful across various industries.
On the other hand, one can argue that if the positive effects of AI are so quick and significant, customers might prefer to continue paying for seats or consumption to capture 100% of the value. We believe that over time, different customers will have different preferences, with some opting for a combination of both models. Therefore, founders need to be flexible with their revenue models and find ways to personalize them based on each customer’s needs.
Up Next: The GCC's AI Gambit To Shape A Future Of Leadership And Innovation
In the next installment of our four-part series, we'll take a deep dive into the region's pioneering journey in Artificial Intelligence, spearheaded by the UAE, to uncover the exciting developments that are shaping the GCC's future as a leader in AI technology.
The UAE stands out as a significant change agent - one which is poised to capture global opportunities and reshape the tech landscape, thanks to its long-term vision and commitment over the years to research, planning, collaboration and innovation. Recent initiatives and partnerships brought forward by Saudi Arabia at national level, including the Saudi Data and AI Authority (SDAIA) further underscores the region’s commitment.
We'll explore the UAE’s strategic initiatives since 2017, including the appointment of the world's first Minister of State for AI. We’ll showcase key government programs, private sector contributions, and academic research driving AI innovation in the region. We'll also examine how strategic collaborations and a comprehensive ecosystem are positioning the GCC as a potential global AI talent hub.